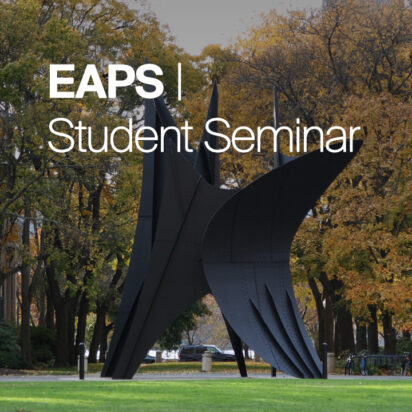
[ESAC Student Seminar] Xin Kai Lee
Date: Thursday, May 9, 2024 Time: 12:00 - 1:00pm Location: 55-110 | MIT Campus, Cambridge, MA“Inductive Bias is All You Need: Physics-Driven, Machine-Augmented Parameterization for Salty Turbulent Mixing in the Upper Ocean”
Ocean models are large and complex numerical solvers of the Navier-Stokes equations in oceanographic settings and can be coupled with atmospheric and land models to produce climate predictions. However, the finest computational grid an ocean model can be run on currently for realistic global simulations has a grid resolution of O(10km). This means that subgrid-scale processes below this grid resolution such as convection and wind-driven mixing in the upper ocean cannot be explicitly resolved by the model. Yet, they have a fundamental impact on the large-scale structure of the ocean by setting the mixed layer depth and thermocline structure, which governs tracer exchange between the boundary layer and the ocean interior. Therefore, parameterizations are required to model the effects of subgrid-scale processes using large-scale variables.
In this work, we present a novel parameterization scheme for the turbulent mixing of the upper ocean using Neural Ordinary Differential Equations (NODEs), taking into account both temperature, salinity, and their nonlinear effects on the equation of state of seawater. The NODEs use training data generated from high fidelity large-eddy simulations (LES) as the ground truth. The model is physics-driven as a local eddy diffusivity closure is used to capture the local mixing effects due to convection and shear. This base closure models wind-only scenarios very well without any neural network augmentation. Then, neural networks are used for free convection cases to augment the model by parameterizing the nonlocal entrainment mixing. By introducing simple and correct predictable inductive biases in the form of known physics and model design, the NODEs are able to parameterize the mixing effects in free convection, and show excellent interpolation capabilities to unseen forcings. The NODEs obey conservation laws and tracer invariance, and are also numerically stable in time. Without the base closure, the NODEs show similar convergence during training, but generalize poorly, give unphysical results, and can be numerically unstable. On the other hand, if we improve the closure by including nonlocal effects due to entrainment, the NODEs train similarly well, but overfit during validation as there is no missing physics left to be learned from the given data. This is because the improved nonlocal closure shows excellent predictive capabilities in all training and validation scenarios without any neural network augmentation. For hybrid models where ODE-based mechanistic dynamics interact with flexible and expressive machine-learned components, if the structural deficiencies are well-defined, there is a greater possibility that what the data-driven component captures are reduced-order dynamics which are physical but missing from the unaugmented model. Understanding the nature of the structural deficiencies will also allow us to build inductive biases into the model design, reducing the data requirement while encouraging robust models which are more likely to train efficiently, generalize more broadly, and give accurate predictions in a wide range of scenarios.
ESAC Student Seminar Series
A forum for students and postdocs to share recent research, hone presentation skills, and build community among peers, sponsored by the EAPS Student Advisory Committee. Open to current EAPS graduate and undergraduate students and postdocs. Typically hosted on Thursdays during the semester, including pizza lunch.
Contact: esac.officers@gmail.com